Project Overview
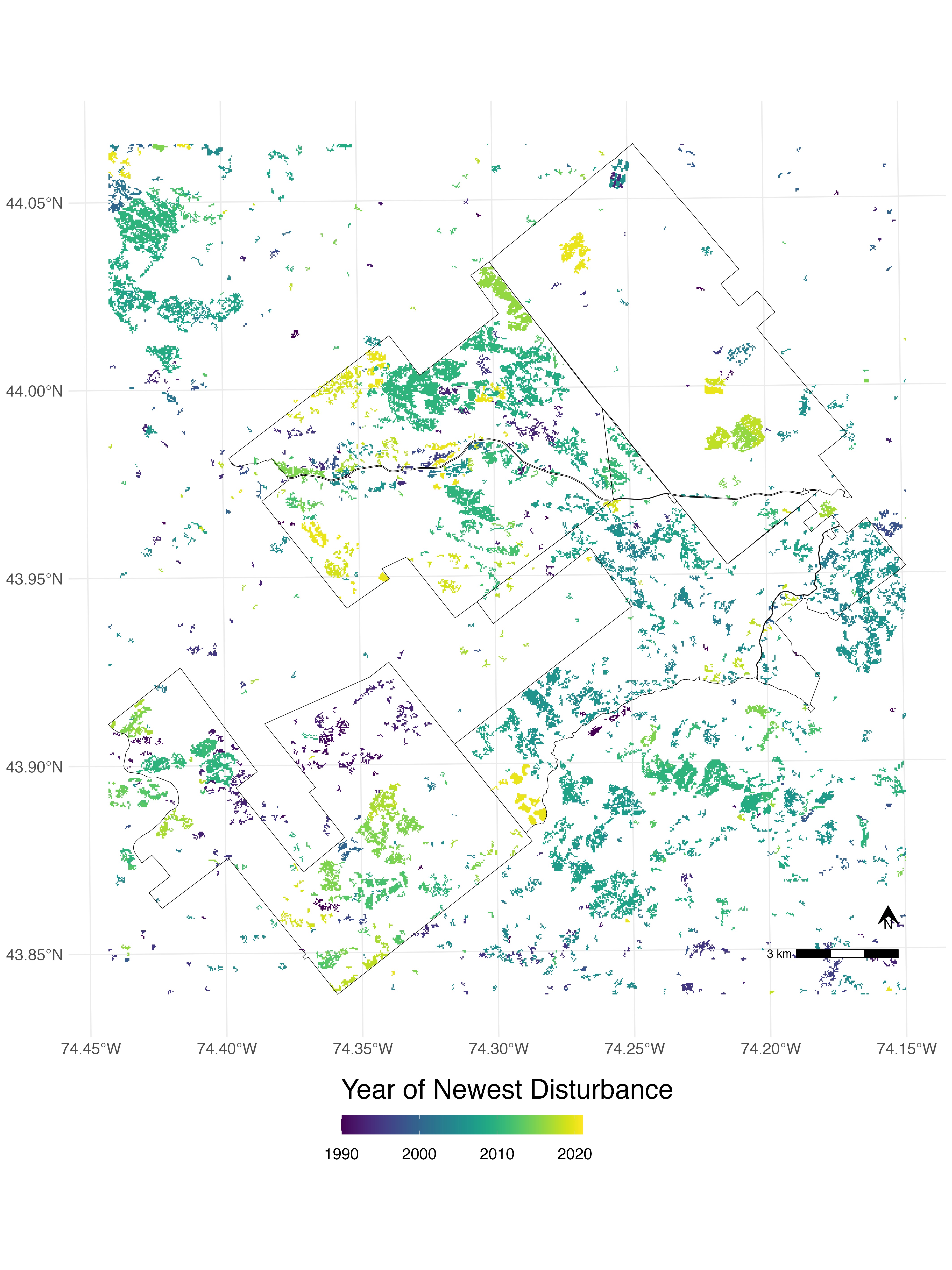
The need for reliable landscape monitoring tools is growing rapidly with increasing attention and reliance on forests as natural climate solutions. However, none of the leading monitoring tools were developed for the forest types and disturbance regimes of the Northern Forest region. To address this problem, our objective was to train a forest change detective using the Landtrendr on Google Earth Engine (LTGEE) platform, which we found was the best performer, by far, yet with ample room for improvement. To this end, we carried out an iterative ‘tuning’ process to improve LT-GEE performance, which we evaluated by comparing outputs against three sources of ‘ground-truth’ data: landowner harvest records/maps, orthoimagery verification (using TimeSync) and field reconnaissance. Overall, through tuning we achieved substantial gains in LT-GEE performance for change detection in the US Northeast, especially for harvest-related disturbances. Based on the best LT-GEE tunings identified among those tested, we generated historical disturbance maps for the entire FEMC coverage area (NY, VT, NH, ME, RI, CT, MA) at 30m annual resolution from 1985-2023. These data products are archived with FEMC along with an online 'user's guide' including code, API scripts and helpful tips. Overall this project has taken the first essential steps in applying the LT-GEE change detection platform for landscape monitoring that supports forest science and stewardship across the US Northeast.
Objectives
Our goal was to develop a version of the LT-GEE algorithm trained specifically for the forest types and disturbance regimes of the FEMC region, that is, the US Northeast. Training this tool relied on existing data-sharing partnerships with working forest landowners, the unique patchwork of private and public forest land in the Adirondacks (NY) region, and the open-source LT-GEE implementation that allows us to carry out algorithm tuning and testing. We used an iterative ‘tuning’ process to calibrate LT-GEE operating parameters to find one or more algorithm configurations that yielded more accurate outputs, based on multiple ground-reference data sources. Supporting objectives included sharing the best-tuned version(s) of the LT-GEE change detection tool that we could produce; generating maps of forest disturbance history (back to 1985) across the NF region; and sharing these maps of disturbance history freely with scientists, practitioners and stakeholders across the region.
Dataset Availability
Downloadable: 1 dataset
See the full list of available dataTags
No tagsStatus - Completed
Start date: 2022-06-01
End date: 2024-05-31
Study Area
